Overview¶
Generalized Operational Perceptron¶
Generalized Operational Perceptron (GOP) is a neuron model taking inspiration from the neuronal activities in the biological learning system of mammals in which each neuron conducts electrical signals over three distinct operations:
- Modification of input signal from the synapse connection in the Dendrites.
- Pooling operation of the modified input signals in the Soma.
- Sending pulses when the pooled potential exceeds a limit in the Axon hillock.
So GOP is designed to also perform 3 distinct operations: nodal, pooling and activation. It has been observed that there are different types of biological neurons which performs differently on the input signal. Thus, to mimic this variety, instead of fixing a single type of operator, each GOP can select its own type of nodal, pooling and activation operator from a library of pre-defined operators. Let’s look at the illustration of the i-th GOP in layer l+1 in a feed-forward network:
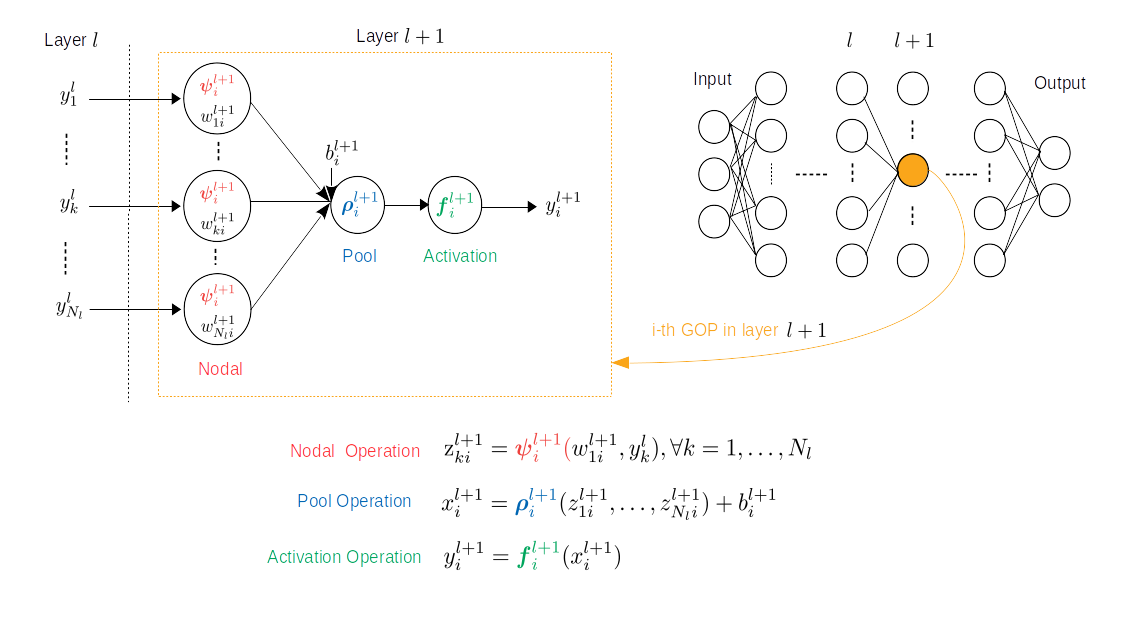
Mathematically, let \(\boldsymbol{\psi}^{l+1}_i\) denote the nodal operator of the i-th GOP in the (l+1)-th layer, nodal operation has the following equation:
As we can see, the nodal operation is applied to each incoming input signal. So if the previous layer generates \(N_l\)-dimensional output vector, the nodal operation is applied to each element in the \(N_l\)-dimensional input vector of the (l+1)-th layer. Each input element has its corresponding tunable weight \(w_{ki}^{l+1}\). Basically, in the nodal operation, GOP manipulates the k-th input signal with parameter \(w_{ki}^{l+1}\) using some kind of transformation. The kind of transformation can be selected (ideally based on optimization) from a set of pre-defined nodal operators.
Similarly, let \(\boldsymbol{\rho}^{l+1}_i\) denote the pooling operator of the i-th GOP in the (l+1)-th layer. The pooling operation is applied on the results of the nodal operation, which is:
The pooling operator has no tunable parameter. The only tunable parameter here is \(b^{l+1}_i\), which represents the bias term. It simply summarizes the \(N_l\) outputs of the nodal operation, offset by the bias term. The functional form of pooling operation is also selected (ideally based on optimization) from a set of pre-defined pooling operators.
Finally, the activation operation is just simply the activation operation in conventional neural network. But instead of fixing a single type of activation, ideally, the network optimization process should select the most suitable one for each GOP. Let \(\boldsymbol{f}^{l+1}_i\) denote the activation operator of i-th GOP in (l+1)-th layer, its equation is:
At the moment, PyGOP supports the following built-in operators which can be specified by names:
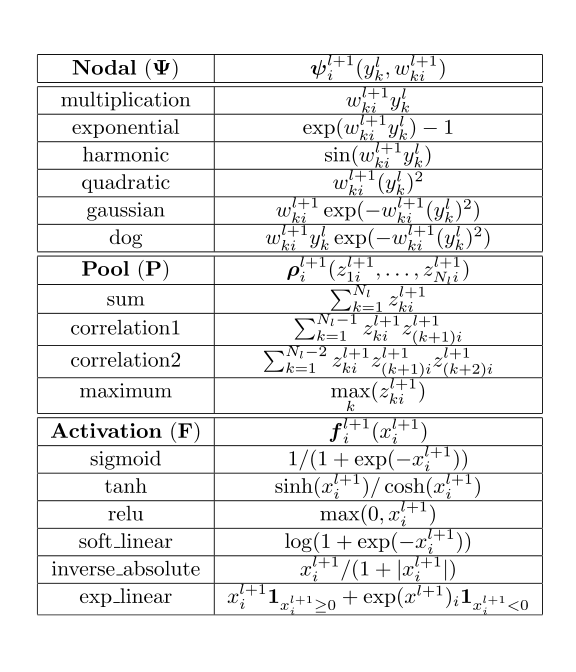
One feature of PyGOP is the support for user-defined operator. Custom Operators documents how to define your own operator.
By allowing greater flexibility in the type of transformation each neuron can have, optimizing a GOP network involves both discrete (the search space of possible combination) and continuous optimization (network’s weights). Even with a small network topology with 200 neurons and the above example library of operators, there are \((6 \times 4 \times 6)^{200} = 144^{200}\) total network configurations. Thus, in order to optimize GOP networks, certain constraints must be made to reduce the amount of computation.
At the moment, PyGOP implements 8 existing algorithms to train GOP networks with different set of constraints:
- Progressive Operational Perceptron (POP)
- Heterogeneous Multilayer Operational Perceptron (HeMLGOP)
- Homogeneous Multilayer Operational Perceptron (HoMLGOP)
- Heterogeneous Multilayer Randomized Network (HeMLRN)
- Homogeneous Multilayer Randomized Network (HoMLRN)
- Fast Progressive Operational Perceptron (POPfast)
- Progressive Operational Perceptron with Memory (POPmem)
For a short description of each algorithm, please go to Algorithms
PyGOP’s Features¶
PyGOP is built on top of Tensorflow and Keras, following a modular structure. The library is distributed via Python Package Index (PyPI) and public repository (github). When designing PyGOP, we aim for the following key features:
- Reproducibility: Existing algorithms optimizing GOP networks at the moment are included in the library, which allows users to benchmark the proposed algorithms. In addition, GOP is implemented as a Keras layer, thus, can be used independently by the users to define any GOP-based networks according to their specification.
- Flexible Computation Environments: PyGOP can run on both CPU and GPU with just one-liner configuration when training the model instance. In addition, the training process can be deployed on a single or multiple machines. In case of distributed computation, our current implementation supports SLURM job scheduler. Due to the modular design, development for a different cluster environment is straightforward.
- Scalability: Beside the distributed computation option that maximizes the degree of parallelization, PyGOP adopts an efficient data feeding mechanism using Python generator that allows users to load and preprocess input data in mibi-batches. This design enables low memory requirement even when processing large datasets.
- Customization: The users have the flexibility to define custom nodal, pooling or activation operators. In addition, custom loss function and custom metrics can be easily defined, or re-weighing the loss values from different classes to tackle the class-imbalance problem can be done in just one line of code.
- Reusability: Saving and Loading a pre-trained model is done in just one line of code. In addition, PyGOP automatically saves checkpoints after each progressive step during the computation and automatically resumes to the last checkpoint when the script is re-launched in case of an interrupt.
Documentation Overview¶
Home¶
- Overview: gives an overview of PyGOP
- Installation gives instruction on how to install PyGOP through pip or source.
- Change Log documents major changes between versions.
User Guide¶
- Quick Start gives a brief introduction on how to use PyGOP interface.
- Data Feeding Mechanism gives instruction on the data feeding mechanism of PyGOP
- Common Interface is dedicated to common parameters and interface shared by all algorithms.
- Computation Environments discusses how to setup parameters related to computation devices and computation environment such as single machine or cluster.
- Algorithms gives brief description of each algorithm and algorithm-specific parameters.
- Customization details how to define custom loss, metrics or operators.
Tutorials¶
- Hand-written Digits Recognition with Mnist dataset provides a working example of using PyGOP to train all algorithms using MNIST dataset available in Keras.
- Face Recognition with CelebA dataset provides another working example of PyGOP to train a face recognition model, including the feature extraction step.
About¶
- Contributions gives instructions on how to contribute to PyGOP.
- License details license statement.